To foster, strengthen and support the development and wide adoption of big data value technologies within an increasingly complex landscape requires an interdisciplinary approach that addresses the multiple elements of big data value. This book captures the early discoveries of the big data value community as an initial set of Elements of Big Data Value. This book arranges these elements into a classification system which is inspired by the periodic table for classifying chemical elements by atomic mass. Within our periodic table we have four groupings (see Fig. 1) containing elements focusing on similar behaviours needed for big data value covering (1) ecosystem, (2) research and innovation, (3) business, policy and societal elements, and (4) emerging elements. As we learn more about how to leverage and derive more value from data, we expect the elements of big data value to be challenged and to evolve as new elements are discovered. Just as the originators of the periodic table left room for new elements, The Periodic Table of the Elements of Big Data Value is open to future contributions.
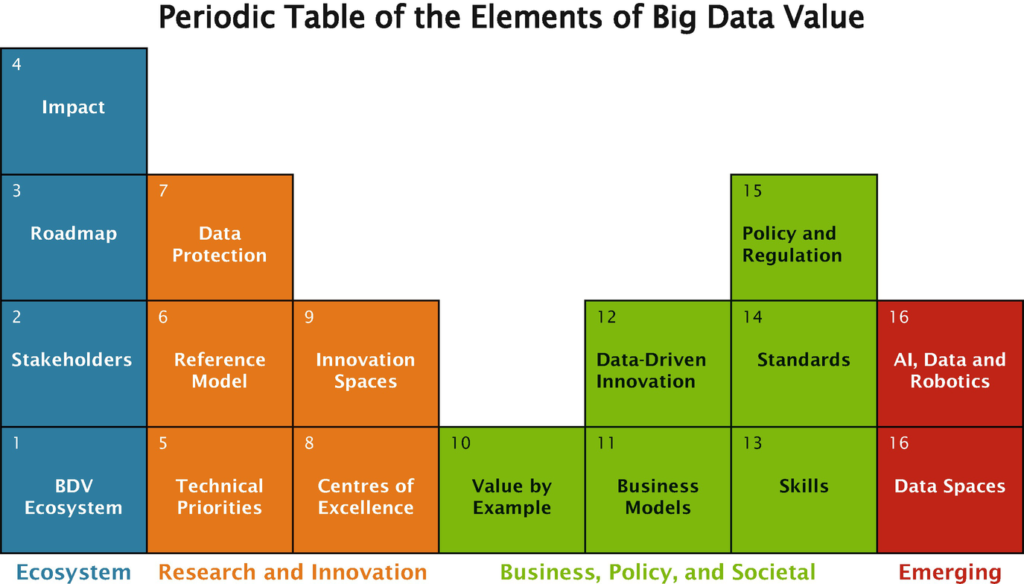
Ecosystem Elements of Big Data Value
The establishment of the big data value ecosystem and promoting its accelerated adoption required a holistic approach to make it strong, vibrant and valuable to its stakeholders. The main elements that needed to be tackled to create and sustain a robust data ecosystem are as follows:
• BDV Ecosystem:
This chapter explores the opportunity to increase the competitiveness of European industries through a data ecosystem by tackling the fundamental elements of big data value, including the ecosystem, research and innovation, business, policy and regulation, and the emerging elements of datadriven AI and common European data spaces.
• Stakeholders:
Chapter “Stakeholder Analysis of Data Ecosystems” discusses a stakeholder analysis concerning data ecosystems and stakeholder relationships within and between different industrial and societal case studies. The stakeholder analysis helps determine how to incentivise stakeholders to participate in the activities of the data ecosystem. Each case study within the analysis focuses on big data practices across a range of industrial sectors to gain an understanding of the economic, legal, social, ethical and political externalities. A horizontal analysis is conducted to identify how positive externalities can be amplified and negative externalities diminished.
• Roadmap:
A roadmap to drive adoption of data value ecosystems is described in Chap. “A Roadmap to Drive Adoption of Data Ecosystems”. Creating a productive ecosystem for big data and driving accelerated adoption requires an interdisciplinary approach addressing a wide range of challenges from access to data and infrastructure, to technical barriers, skills, and policy and regulation. Overcoming these challenges requires collective action from all stakeholders working together in an effective, holistic and coherent manner. To this end, the Big Data Value Public-Private Partnership was established to develop the European data ecosystem and enable data-driven digital transformation, delivering maximum economic and societal benefit.
• Impact:
Chapter “Achievements and Impact of the Big Data Value PublicPrivate Partnership: The Story so Far” details the impact of the Big Data Value Public-Private Partnership, which plays a central role in the implementation of the European Data Economy. The chapter provides an overview of the partnership and its objectives, together with an in-depth analysis of the impact of the PPP.
Research and Innovation Elements of Big Data Value
New technical concepts will emerge for data collection, processing, storing, analysing, handling, visualisation and, most importantly, usage, and new data driven innovations will be created using them. The key research and innovation elements of big data value are as follows:
• Technical Priorities:
Chapter “Technical Research Priorities for Big Data” details the technical priorities for big data value covering key aspects such as real-time analytics, low latency and scalability in processing data, new and rich user interfaces, interacting with and linking data, information and content, all of which have to be developed to open up new opportunities and to sustain or develop competitive advantages. As well as having agreed approaches, the interoperability of datasets and data-driven solutions is essential to ensure broad adoption within and across sectors.
• Reference Model:
Chapter “A Reference Model for Big Data Technologies” describes the Big Data Value Reference Model, which has been developed with input from technical experts and stakeholders along the whole big data value chain. The BDV Reference model serves as a common reference framework to locate data technologies on the overall IT stack. It addresses the main concerns and aspects to be considered for big data value systems.
• Data Protection:
Data Protection and Data Technologies are the focus of Chap. “Data Protection in the Era of Artificial Intelligence: Trends, Existing Solutions and Recommendations for Privacy-Preserving Technologies”, where advances in privacy-preserving technologies are aimed at building privacy-by design from the start into the back-end and front-end of digital services. They make sure that data-related risks are mitigated both at design time and run time, and they ensure that data architectures are safe and secure. The chapter discusses recent trends in the development of tools and technologies that facilitate secure and trustworthy data analytics.
• Centres of Excellence:
Chapter “A Best Practice Framework for Centres of Excellence in Big Data and Artificial Intelligence” presents a best practice framework for Centres of Excellence for Big Data and AI. Within universities, academic departments and schools, it often works towards the establishment of a special-purpose organizational unit within a national system of research and education that provides leadership in research, innovation and training for Big Data and AI technologies. Centres of Excellence can serve as a common practice for the accumulation and creation of knowledge that addresses the scientific challenges of Big Data and AI, opens new avenues of innovation in collaboration with industry, engages in the policy debates, and informs the public about the externalities of technological advances.
• Innovation Spaces:
Within the European data ecosystem, cross-organisational and cross-sectorial experimentation and innovation environments play a central role. Chapter “Data Innovation Spaces” describes the European Innovation Spaces, which are the main elements to ensure that research on big data value technologies and novel applications can be quickly tested, piloted and exploited to the maximum benefit of all the stakeholders.
Business, Policy and Societal Elements of Big Data Value
Big data is an economic and societal asset that has significant potential for the economy and society. New sustainable economic models within a policy environment that respects data owners and individuals are needed to the deliver value from big data. Critical elements of big data value for business and policy are as follows:
• Value Creation:
Chapter “Big Data Value Creation by Example” provides a collection of stories showing concrete examples of the value created thanks to big data value technologies. These novel solutions have been developed and validated by stakeholders in the big data value ecosystems and provide proof points of how data can drive innovation across industries to transform business practices and society. Meanwhile, start-ups are working at the confluence of emerging data sources (e.g. IoT, DNA, high-definition images, satellite data) and new or revisited processing paradigms (e.g. Edge computing, blockchain, machine learning) to tackle new use cases and provide disruptive solutions.
• Business Models:
Chapter “Business Models and Ecosystem for Big Data” explores new data-driven business models as ways to generate value for companies along the value chain, regardless of sector or domain: optimising and improving the core business; selling data services; and, perhaps most importantly, creating entirely new business models and business development. Identifying sustainable business models and ecosystems in and across sectors and platforms will be an important challenge. In particular, many SMEs that are now involved in highly specific or niche roles will need support to help them align and adapt to new value chain opportunities.
• Data-Driven Innovation:
Chapter “Innovation in Times of Big Data and AI: Introducing the Data-Driven Innovation (DDI) Framework” introduces the DataDriven Innovation (DDI) Framework to support the process of identifying and scoping big data value. The framework guides start-ups, entrepreneurs and established companies alike in scoping promising data business opportunities by analysing the dynamics of both supply and demand.
• Skills:
Chapter “Recognition of Formal and Non-formal Training in Data Science” covers the data skills challenge to ensure the availability of appropriately skilled people who have an excellent grasp of the best practices and technologies for delivering big data value solutions. Promoting the “transparency and recognition of skills and qualifications” is particularly relevant to the task of recognizing both formal and informal data science training, and consequently the challenge will be to provide a framework in order to validate these skills.
• Standards:
Chapter “The Road to Big Data Standardisation” covers the critical topic of standards within the area of big data where the use of standardised services and products is needed to effectively drive the adoption of common data solutions and services around the world. This chapter provides an overview of the key standardisation activities within the European Union and the current status and future trends of big data standardisation.
• Policy and Regulation:
Chapter “The Role of Data Regulation in Shaping AI: An Overview of Challenges and Recommendations for SMEs” engages in the debate on data ownership and usage, data protection and privacy, security, liability, cybercrime and Intellectual Property Rights (IPR). A necessary first step is to frame this policy and regulatory debate about the non-technical aspects of big data value creation as part of the data-driven economy. These issues need to be resolved to remove the barriers to adoption. Favourable European regulatory environments are required to facilitate the development of a genuine pan-European big data market. For an accelerated adoption of big data, it is critical to increase awareness of the benefits and the value that big data offers, and to understand the obstacles to building solutions and putting them into practice.
Emerging Elements of Big Data Value
Artificial Intelligence (AI) has tremendous potential to benefit citizens, economy and society. From a big data value perspective, AI techniques can extract new value from data to enable data-driven systems that in turn enable machines and people with digital capabilities, such as perception, reasoning, learning and even autonomous decision-making. Data ecosystems are an essential driver for data-driven AI to exploit the continued growth of data. Developing both of these elements together is critical to maximising the future potential of big data value:
• Artificial Intelligence, Data and Robotics:
To maximise the potential of AI, a solid foundation is needed for successfully deploying AI solutions. To this end, Chap. “Data Economy 2.0: From Big Data Value to AI Value and a European Data Space” details the European AI, Data and Robotics Framework (Zillner et al. 2020), which represents the legal and societal fabric that underpins the impact of AI on stakeholders and users of the products and services that businesses will provide. The AI, Data and Robotics Innovation Ecosystem Enablers represent essential ingredients for significant innovation and deployment to take place within this framework. Finally, Cross Sectorial AI, Data and Robotics Technology Enablers are needed to provide the core technical competencies that are essential for the development of data-driven AI systems.
• Data Spaces:
As part of the continued development of the European Big Data Value Ecosystem, Chap. “Data Economy 2.0: From Big Data Value to AI Value and a European Data Space” describes common European data spaces which will be established to ensure that more data becomes available for use in the economy and society while keeping companies and individuals who generate data in control. These data spaces (in both a technical Curry 2020] and regulatory [European Commission 2018] sense) will be critical to fuelling data-driven AI innovations.
Excerpt from: Curry E. et al. (2021) The European Big Data Value Ecosystem. In: Curry E., Metzger A., Zillner S., Pazzaglia JC., García Robles A. (eds) The Elements of Big Data Value. Springer, Cham. https://doi.org/10.1007/978-3-030-68176-0_1
References:
- Zillner, S., Bisset, D., Milano, M., Curry, E., Hahn, T., Lafrenz, R., et al. (2020). Strategic research, innovation and deployment agenda – AI, data and robotics partnership. Third Release (3rd). Brussels: BDVA, euRobotics, ELLIS, EurAI and CLAIRE.